- Published on
How to Form a Hypothesis Statement
- Authors
- Name
- Tony
- @shyeditor
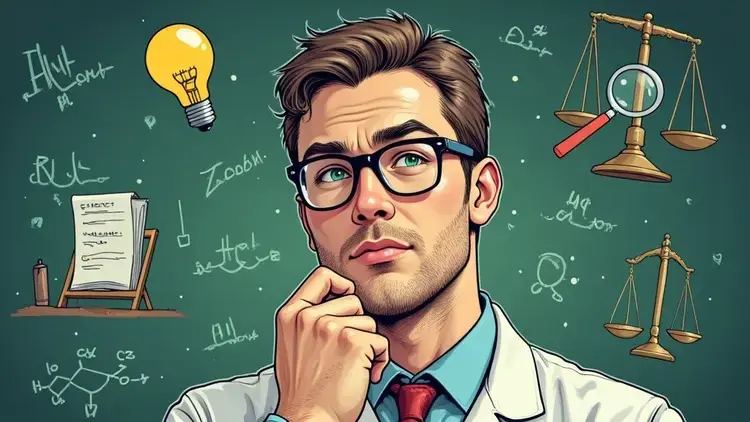
Understanding What Makes Hypotheses Work
Creating an effective hypothesis requires more than just making an educated guess. A strong hypothesis serves as the foundation of good research by providing a testable prediction based on careful observation and existing knowledge. When crafted thoughtfully, your hypothesis acts like a roadmap that guides your entire research process and helps ensure your findings contribute meaningful insights to your field.
Essential Elements of a Strong Hypothesis
Several key components work together to create a hypothesis that can effectively drive research:
Clarity and Specificity: Vague statements limit research value. For example, rather than saying "Music affects mood," a better hypothesis would be "Listening to classical music reduces anxiety levels in college students." This precise focus enables meaningful testing.
Testability: Your hypothesis must be something you can actually verify through research methods. Claims like "invisible unicorns exist in my garden" can't be tested scientifically. Focus on relationships and outcomes you can measure.
Falsifiability: A good hypothesis must be able to be proven wrong. For instance, "All swans are white" is falsifiable because finding just one black swan would disprove it. This openness to being disproven is essential for scientific rigor.
Research Foundation: Connect your hypothesis to previous studies and established theories in your field. This grounds your work in existing knowledge while showing how it builds upon prior research.
Meaningful Focus: Choose research questions that matter. Your hypothesis should address significant gaps in understanding or practical problems rather than trivial issues.
Distinguishing Between Null and Alternative Hypotheses
Understanding how to work with null and alternative hypotheses is key for proper testing. The null hypothesis (H0) states no relationship exists between your variables, while the alternative hypothesis (H1 or Ha) proposes that a relationship does exist.
Consider this example of plant fertilizer research:
- Null Hypothesis (H0): The new fertilizer shows no effect on plant growth
- Alternative Hypothesis (H1): The new fertilizer increases plant growth
This framework lets you analyze data systematically to determine if you have enough evidence to reject the null hypothesis. Remember that failing to reject the null doesn't prove it true - it simply means your data doesn't support the alternative hypothesis strongly enough.
Avoiding Common Pitfalls
Watch out for these frequent mistakes when developing hypotheses:
Correlation vs. Causation: Just because two things happen together doesn't mean one causes the other. Ice cream sales and crime rates might both rise in summer, but that doesn't mean ice cream causes crime. Be clear about what relationship you're actually testing.
Excess Complexity: While some research involves multiple variables, trying to test too many relationships at once makes analysis difficult. Break complex questions into smaller, focused hypotheses you can test effectively.
Unclear Definitions: Define exactly what you're measuring. In a plant growth study, specify whether you're tracking height, weight, leaf count, or other metrics. Clear operational definitions ensure reliable data collection.
By paying attention to these key elements and common challenges, you'll create hypotheses that effectively guide your research and lead to meaningful results. This foundation in hypothesis development helps ensure your work contributes valuable insights to your field.
Crafting Null Hypotheses That Set You Up for Success
A well-designed null hypothesis forms the essential starting point for effective statistical testing. Much like establishing baseline measurements before an experiment, the null hypothesis provides a clear reference point against which you can evaluate your research findings. It begins with the simple assumption that no relationship exists between your variables - not because we expect this to be true, but because it gives us a statistical baseline for comparison.
Defining the Role of the Null Hypothesis
The null hypothesis (H0) works similarly to the presumption of innocence in a court trial. Just as defendants are presumed innocent until proven guilty beyond reasonable doubt, we assume no relationship between variables until we have strong statistical evidence suggesting otherwise. This systematic approach helps prevent rushed or unfounded conclusions.
For instance, when studying whether a new teaching method impacts student performance, your null hypothesis might state: "There is no significant difference in test scores between students taught with the new method versus the traditional method." This gives you a clear benchmark for statistical comparison, in contrast to the alternative hypothesis which would propose that a difference exists.
Constructing Effective Null Hypotheses
Creating a strong null hypothesis requires careful attention to three key elements:
Specificity: Write your hypothesis with precise, measurable terms. Instead of saying "The new drug has no effect," specify "The new drug shows no statistically significant effect on blood pressure compared to the placebo."
Testability: Make sure you can evaluate your hypothesis through statistical analysis. Your variables must be measurable and the proposed relationship (or lack thereof) should be something you can test with available statistical methods.
Focus: Keep your null hypothesis directly aligned with your core research question. A misaligned hypothesis will lead you down unproductive paths and waste valuable research time.
Assessing Your Null Hypothesis: Ready for Testing?
Before moving forward with data collection, evaluate your null hypothesis against these key criteria:
Clear Connection to Research Question: Does your null hypothesis directly address what you're trying to learn? If not, revise until it does.
Measurable Variables: Have you clearly defined how you'll measure each variable? Without specific measurements, you can't properly test your hypothesis.
Statistical Testability: Can you identify which statistical test will help you analyze your results? This ensures you have a valid way to determine significance.
Think of a well-crafted null hypothesis like laying the foundation for a house - it needs to be solid and level before you can build anything on top of it. By focusing on clarity, measurability, and alignment with your research goals, you create the conditions for meaningful analysis and reliable conclusions. This careful preparation helps ensure your research will produce valuable insights that contribute to your field. In the next section, we'll examine how to develop the alternative hypothesis that pairs with your null hypothesis.
Developing Alternative Hypotheses
Once you've created a solid null hypothesis, the next key step in hypothesis formation is developing its counterpart - the alternative hypothesis. This statement captures what you expect to find if your research reveals a connection between your study variables. A thoughtfully constructed alternative hypothesis provides essential guidance for your research approach and helps make sense of your findings.
Structuring Your Alternative Hypothesis
The alternative hypothesis (H1 or Ha) suggests that your variables are related in some way. Your hypothesis structure needs to clearly outline this proposed relationship, and researchers typically choose between two main approaches: directional and non-directional hypotheses.
Directional Hypothesis: This format predicts exactly how variables affect each other - for example, "Increased daily exercise leads to a decrease in resting heart rate." Researchers use directional hypotheses when existing studies or strong theories point to a specific type of relationship.
Non-Directional Hypothesis: This simpler format just states that variables are connected, without specifying how. For instance: "There is a relationship between caffeine consumption and sleep quality." This works well for exploring new research areas or when current evidence doesn't suggest a clear direction. The choice between approaches depends on your background knowledge and research goals.
Illustrative Examples: From Theory to Hypothesis
To see how this works in practice, let's look at a study on social media's effects on teenage self-esteem:
- Null Hypothesis (H0): There is no relationship between social media usage and self-esteem in teenagers.
- Directional Alternative Hypothesis (H1): Increased social media usage is associated with lower self-esteem in teenagers.
- Non-Directional Alternative Hypothesis (H1): There is a relationship between social media usage and self-esteem in teenagers.
Here's another example examining a new blood pressure medication:
- Null Hypothesis (H0): The new medication has no effect on blood pressure.
- Directional Alternative Hypothesis (H1): The new medication lowers blood pressure.
- Non-Directional Alternative Hypothesis (H1): The new medication affects blood pressure.
These examples show how alternative hypotheses propose relationships between variables, while null hypotheses state no relationship exists. Your choice between directional and non-directional forms should reflect current research and your specific research question. Creating a clear, testable alternative hypothesis helps ensure your research makes meaningful contributions to your field's knowledge base.
Making the 'Simple vs. Composite' Decision
Once you've established your null and alternative hypotheses, you'll need to make an important choice between using a simple or composite hypothesis. This decision shapes how you'll conduct your statistical tests and interpret the results. Let's explore the key differences between these types of hypotheses and how to choose the right one for your research.
Understanding Simple Hypotheses
A simple hypothesis states one specific value for what you're measuring in a population. It's like aiming for an exact bullseye - there's only one correct outcome. For instance, when studying sunflower heights in a field, a simple hypothesis might state "The average height of sunflowers in this field is exactly 6 feet." This precise nature makes simple hypotheses straightforward to test since you have a clear benchmark for comparison.
Exploring Composite Hypotheses
On the other hand, a composite hypothesis works with a range of possible values rather than just one. Think of it as accepting any hit within a larger target area, not just the bullseye. Using our sunflower example, a composite hypothesis might say "The average height of sunflowers in this field is greater than 5 feet." This allows for any height above 5 feet to support your hypothesis. While this flexibility can be useful, it sometimes requires more complex statistical analysis.
Choosing the Right Hypothesis
Your choice between simple and composite depends on your research goals and available information. Simple hypotheses work best when testing against specific, known values. For example, if you're testing a new drug dosage where precision is critical, a simple hypothesis focusing on an exact target dose makes sense.
But when you're exploring broader patterns or don't need such precision, composite hypotheses offer helpful flexibility. Consider studying customer satisfaction - a composite hypothesis like "Customer satisfaction scores are greater than 7 out of 10" makes more sense than trying to predict an exact score.
Real-World Examples: Simple vs. Composite in Action
Here are some concrete examples to illustrate the difference:
Simple Hypothesis Example: A light bulb manufacturer claims their bulbs last exactly 1,000 hours. This precise claim needs a simple hypothesis for testing.
Composite Hypothesis Example: A teacher believes their new teaching method improves test scores, without specifying exactly how much improvement to expect. This less precise prediction calls for a composite hypothesis.
Quality control offers another clear comparison:
Simple Hypothesis Example: A bottling machine should fill containers with exactly 16 ounces. Quality testing this precise volume uses a simple hypothesis.
Composite Hypothesis Example: A nutrition study suggests that vitamin C intake above a certain threshold boosts immunity, without specifying the exact amount needed. This broader claim requires a composite hypothesis.
These examples show how your choice of hypothesis type should match both your research question's specificity and what you're measuring. Understanding these distinctions helps you create hypothesis statements that align with your research goals and lead to meaningful results.
Connecting Hypotheses to Statistical Reality
Creating a solid hypothesis statement is just the beginning. The real challenge lies in connecting your theoretical predictions to actual statistical analysis. This section explores how to craft hypothesis statements that work seamlessly with statistical testing methods to produce meaningful results.
Aligning Your Hypothesis With Statistical Methods
The statistical test you choose must match both your data type and hypothesis goals. For instance, when comparing men's and women's average heights, a t-test makes sense. But if you want to understand how study time affects exam scores, correlation analysis would be more appropriate. Each hypothesis requires careful consideration of the statistical method that will best validate or disprove it.
Significance Levels and P-Values: Interpreting the Evidence
Most researchers use a significance level (alpha) of 0.05, meaning there's a 5% chance of incorrectly rejecting a true null hypothesis. The p-value you get from your analysis shows how likely your results would be if the null hypothesis were true. When your p-value falls below your chosen significance level, you can reject the null hypothesis in favor of the alternative.
For example, if you get a p-value of 0.03, this means that if you ran your study 100 times when the null hypothesis was actually true, you'd only see results this extreme 3 times. Such a low probability suggests strong evidence against the null hypothesis.
Adapting Hypothesis Testing Across Different Fields
While the basic principles stay the same, different fields apply hypothesis testing in unique ways. Medical research often uses stricter significance levels like 0.01 because of serious health implications. Many fields now focus on effect size alongside statistical significance to understand practical impact. Each discipline adapts these tools to match its specific needs.
Common Pitfalls and How to Avoid Them
Watch out for these frequent mistakes when connecting hypotheses to statistics:
Misinterpreting the P-Value: Remember - the p-value is not the probability that your null hypothesis is true. It only shows how likely your data would be if the null hypothesis were true. This subtle difference matters greatly for proper interpretation.
Ignoring Effect Size: Statistical significance doesn't always mean practical importance. A tiny effect might not matter in real life, even if it's statistically significant. Always consider both the statistical and practical impact of your findings.
Multiple Comparisons: Running many statistical tests on one dataset increases the risk of false positives. Use methods like the Bonferroni correction to account for multiple comparisons and maintain accurate results.
By understanding these concepts and avoiding common errors, you can create meaningful connections between your hypotheses and statistical analysis. This careful approach ensures your research produces reliable insights that advance knowledge in your field. Remember that forming an effective hypothesis involves not just writing it well, but also testing it properly through statistics.
From Theory to Practice: Making Your Hypotheses Work
A well-crafted hypothesis forms the foundation of effective research. Let's explore practical ways to create hypothesis statements that will guide your research successfully, with real examples and actionable tips.
Avoiding Common Hypothesis Pitfalls
When developing your hypothesis, watch out for several common mistakes that can derail your research. One frequent error is mistaking correlation for causation. For example, while ice cream sales and crime rates both increase during summer months, one doesn't cause the other - they simply share a seasonal pattern. Another pitfall is creating overly complex hypotheses with too many variables, which makes testing and analysis difficult. Keep your focus simple and specific. Also, be precise in your definitions - if you're studying "plant growth," clearly specify whether you're measuring height, leaf count, or another specific metric.
Adapting Your Hypothesis: Maintaining Scientific Integrity
Research often reveals unexpected findings that might tempt you to change your hypothesis. While it's okay to refine your approach based on preliminary results, changing your hypothesis after collecting data to match your findings compromises scientific integrity. Instead, document any modifications openly and explain your reasoning clearly. This balance between flexibility and careful documentation helps maintain the quality of your research.
Case Studies: Deconstructing Effective Hypotheses
Looking at real research examples shows what makes a strong hypothesis work. Take a medical study examining smoking and lung cancer. A good hypothesis might state: "Individuals who smoke a pack of cigarettes daily have a significantly higher risk of developing lung cancer compared to non-smokers." This works because it's specific and testable. Compare this to a weak hypothesis like "Smoking is bad" - too vague to test effectively. In social science research, you might see: "Exposure to violent video games increases aggression in adolescents." This clearly predicts a measurable outcome that can be tested.
Practical Checklists and Verification Tools
To ensure your hypothesis is ready for testing, use a systematic approach. You might find these Examples of Hypothesis in Research helpful. Here's a basic checklist to evaluate your hypothesis:
- Specificity: Are your variables and predicted relationships clearly defined?
- Testability: Can you verify or disprove your hypothesis through experiments?
- Relevance: Does your hypothesis address an important research question?
- Clarity: Is your wording precise and unambiguous?
You can improve your hypothesis writing with Shy Editor, which offers grammar checking and style suggestions to help clarify your research statements. This tool helps ensure your hypothesis is both scientifically sound and clearly expressed.