- Published on
Examples of Hypothesis in Research
- Authors
- Name
- Tony
- @shyeditor
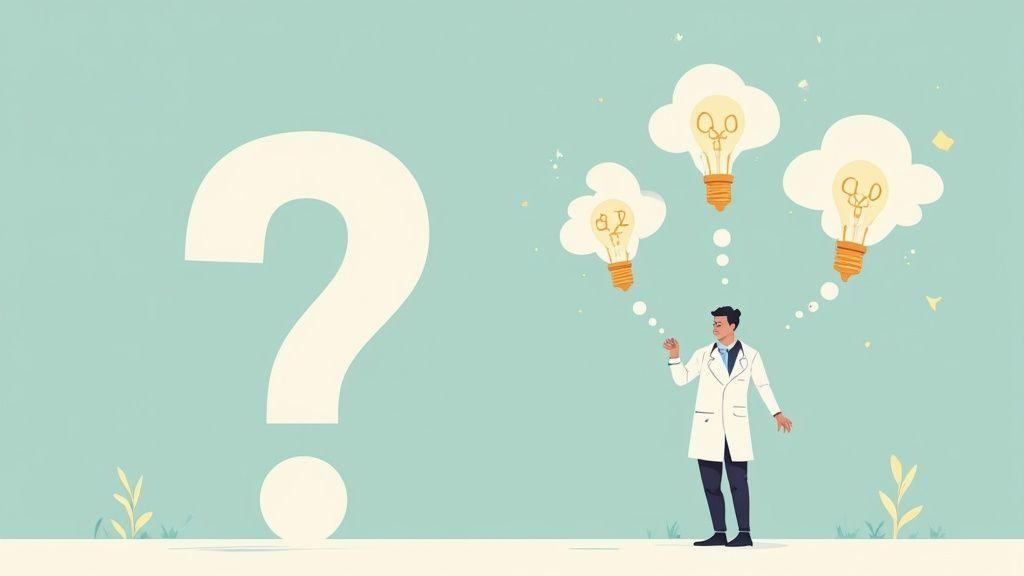
Introduction to Research Hypotheses
A research hypothesis forms the bedrock of any scientific investigation. It's essentially a testable prediction about the connection between two or more variables, an informed guess that guides the research process. This means that a well-constructed hypothesis is essential for obtaining useful and relevant results. Numerous examples of hypotheses across diverse research fields highlight their importance in expanding our knowledge.
The Importance of Hypotheses in Research
Hypotheses are more than just starting points; they play several key roles. A well-crafted hypothesis, for instance, provides a clear direction for the study, preventing it from becoming too broad or unfocused. It also informs the selection of appropriate methods for gathering and analyzing data. This ensures the study effectively tests the specific prediction outlined in the hypothesis. Moreover, hypotheses offer a structured framework for interpreting findings, linking the results back to the initial prediction. This, in turn, reinforces the research process and leads to a deeper understanding of the subject matter.
Hypotheses as a Roadmap for Research
A hypothesis serves as a roadmap for the entire research project. It shapes the study design, influencing choices about participant selection, data collection techniques, and the statistical analyses used. For example, a hypothesis suggesting a positive correlation between exercise and stress reduction would likely involve measuring exercise levels and stress indicators in a participant group. Further, a hypothesis aids in the interpretation of the study's outcomes. If the results reveal a correlation between increased exercise and lower stress levels, it supports the initial hypothesis. However, if no relationship or a negative correlation is discovered, the hypothesis may need revision or rejection. This iterative process of hypothesis testing is essential for refining our knowledge and ensuring that research remains rigorous and contributes meaningfully to existing knowledge. Therefore, grasping the nuances of developing and testing a research hypothesis is fundamental for any researcher. Examining various examples of hypotheses across different research domains can provide practical insights into their application.
Types of Research Hypotheses
Understanding the different types of research hypotheses is essential for designing effective studies. Just as a carpenter uses different tools for various tasks, researchers utilize different types of hypotheses to investigate specific relationships between variables. This selection process ensures the research question is addressed precisely and comprehensively. Examining real-world examples across these various hypothesis types illuminates their practical application.
Simple Hypotheses
A simple hypothesis predicts the relationship between a single independent variable and a single dependent variable. This is the most basic type, focusing on a direct cause-and-effect link. For example, a simple hypothesis might predict that increasing daily water intake (the independent variable) leads to improved skin hydration (the dependent variable). This clear and uncomplicated relationship makes simple hypotheses straightforward to test and interpret. They often serve as the foundation for more complex research inquiries.
Complex Hypotheses
Complex hypotheses, in contrast, predict the relationship between two or more independent variables and two or more dependent variables. These hypotheses reflect the interconnected nature of factors within real-world phenomena. For example, a complex hypothesis might propose that a combination of regular exercise and a balanced diet (independent variables) leads to both weight loss and improved cardiovascular health (dependent variables). Untangling the relationships between these multiple variables requires more intricate research designs.
Directional Hypotheses
A directional hypothesis specifies the nature of the relationship between variables. It predicts not only the existence of a relationship but also its direction. For instance, a directional hypothesis might suggest that as temperature rises (independent variable), ice cream sales also increase (dependent variable). This anticipates a positive correlation, providing a more precise prediction than a non-directional hypothesis. This specificity directly impacts the choice of statistical tests used for data analysis.
Non-Directional Hypotheses
Unlike directional hypotheses, non-directional hypotheses simply predict the existence of a relationship between variables without specifying the direction. They acknowledge a potential link but avoid assumptions about its nature. An example of a non-directional hypothesis would be stating a relationship exists between playing video games and academic performance without predicting whether the relationship is positive or negative. This approach is common in exploratory research where the direction of the relationship is unclear. Further research, perhaps involving directional hypotheses, would then be needed.
Null and Alternative Hypotheses
Finally, null and alternative hypotheses are essential for statistical hypothesis testing. A null hypothesis proposes there is no relationship between the variables, acting as a default assumption researchers aim to disprove. Conversely, the alternative hypothesis proposes there is a relationship. For example, a null hypothesis might state there is no difference in test scores between students who study in silence and those who listen to music. The alternative hypothesis would propose a difference exists. These two hypotheses form the basis of numerous statistical tests, enabling researchers to assess whether the observed data provides sufficient evidence to reject the null hypothesis in favor of the alternative. This process is a cornerstone of generating robust examples of hypotheses in research.
How to Formulate a Hypothesis
Formulating a solid hypothesis is essential for conducting effective research. It's not merely a guess but a testable prediction grounded in existing knowledge and observations. Much like constructing a building requires a detailed blueprint, research requires a strong hypothesis before data collection begins. This section offers practical guidance for developing effective hypotheses, illustrated with examples from actual research.
Defining Your Research Question
The first step in formulating a hypothesis is to clearly identify your research question. This question should be precise, focused, and address a gap in current knowledge. For instance, instead of asking broadly "Does music affect people?" a more focused question might be "Does listening to classical music enhance memory recall in adults?" This refined question sets the stage for a testable hypothesis. Our guide on how to write a research paper offers more in-depth guidance on structuring your research.
From Question to Hypothesis
Once you have a well-defined research question, you can begin formulating your hypothesis. This involves proposing a specific relationship between the variables identified in your research question. Continuing with the classical music example, a plausible hypothesis might be: "Listening to classical music improves memory recall in adults." This statement presents a clear prediction that can be investigated through research.
Specificity and Testability
A robust hypothesis is both specific and testable. It should clearly define the variables and the predicted relationship between them. Testability is paramount, meaning the hypothesis must be framed in a way that allows for data collection and analysis to either support or refute the prediction. For example, a hypothesis like "Classical music is better" isn't testable because "better" is subjective. A testable hypothesis must involve measurable variables.
Considering Different Hypothesis Types
As discussed earlier, various types of hypotheses exist, including null and alternative, directional and non-directional, and simple and complex hypotheses. Your choice depends on your research question. For example, a directional hypothesis predicts a specific direction in the relationship between variables (e.g., "Increased screen time reduces sleep quality"), whereas a non-directional hypothesis predicts a relationship without specifying the direction (e.g., "Screen time and sleep quality are related"). Understanding these different types allows you to select the most appropriate one for your specific research.
Refining Your Hypothesis
After developing your initial hypothesis, refinement is crucial for clarity, conciseness, and accuracy. The hypothesis should be free of jargon and ambiguity. This process often involves consulting existing research and seeking input from experts. The goal is to develop a hypothesis strong enough to guide your research effectively and contribute meaningfully to the existing body of knowledge. By carefully following these steps, researchers create hypotheses that serve as a compass for their investigation, ensuring the project stays aligned with the initial predictions. This process increases the potential for generating impactful insights from collected data, leading to valuable contributions within the field and providing strong examples of hypotheses in research.
Real-world Hypothesis Examples
While understanding how to construct a hypothesis is fundamental, practical examples can solidify this understanding. This section provides real-world examples across various fields, illustrating different types and applications of hypotheses, bridging the gap between theory and practice.
Examples in Psychology
Psychology provides a multitude of examples, often investigating complex human behaviors. A researcher might hypothesize that "Exposure to violent video games increases aggression in adolescents." This directional hypothesis predicts a specific relationship between video game exposure and aggression. A non-directional example would be "There is a relationship between sleep deprivation and cognitive performance," simply stating a relationship exists without specifying its direction. These diverse examples showcase the versatility of hypotheses in psychological research.
Examples in Marketing
Marketing research relies on hypotheses to understand consumer behavior and optimize marketing strategies. A company might hypothesize that "Introducing a loyalty program will increase customer retention." This testable prediction can be evaluated by comparing customer retention before and after the program's launch. Another example might involve A/B testing different website designs with the hypothesis "Website version A will result in a higher conversion rate than website version B." This type of research provides direct feedback on the effectiveness of marketing strategies.
Examples in Medicine
Medical research heavily depends on hypotheses to evaluate treatment efficacy and understand disease mechanisms. A common example involves drug trials where a researcher might hypothesize "Drug X will reduce blood pressure more effectively than Drug Y in hypertensive patients." This is a comparative hypothesis directly contrasting two treatments. Another example could be a null hypothesis stating, "There is no difference in survival rates between patients receiving chemotherapy and patients receiving a new experimental treatment," forming a baseline for evaluating the new treatment's potential benefits.
Examples in Environmental Science
Environmental science utilizes hypotheses to study ecosystems, pollution, and climate change. A researcher might hypothesize that "Increased carbon dioxide emissions contribute to rising global temperatures." This causal hypothesis proposes a cause-and-effect relationship. Another example focusing on conservation might hypothesize that "Implementing reforestation programs will increase biodiversity in degraded areas." These examples illustrate how hypotheses inform our understanding of and responses to environmental challenges.
Examples in Sociology
Sociological research uses hypotheses to study social structures, interactions, and change. For instance, a sociologist might hypothesize that "Higher levels of education are associated with increased social mobility," proposing a correlation between education and social status. Another example might explore social inequality, hypothesizing that "Income disparity contributes to higher crime rates in urban areas." This shows how hypotheses investigate complex social issues and their underlying causes.
Furthering this, the use of null and alternative hypotheses adds another layer of investigation. A null hypothesis might state, "There is no difference in voting patterns between different age groups," while the alternative hypothesis would propose a difference exists. This framework allows for statistical analysis to determine the likelihood of the observed data if the null hypothesis were true. By reviewing these real-world examples, we gain a deeper appreciation for the importance of hypotheses across disciplines. They are not just starting points but crucial tools for framing research questions, guiding data collection, and interpreting results, ultimately advancing knowledge.
Common Mistakes to Avoid
Having explored real-world examples, it's equally vital to understand common pitfalls that can weaken a hypothesis and, therefore, the research. Just as a chef must carefully balance ingredients, researchers must meticulously craft their hypotheses to ensure they are robust and contribute meaningfully to the investigation.
Lack of Clarity and Specificity
A frequent mistake is creating a vague or ambiguous hypothesis, which hinders testing and interpretation. For example, "Social media impacts people" is too broad. It lacks specifics about the type of impact, which aspects of social media, and whom it impacts. A stronger hypothesis would be "Excessive Instagram use increases body image dissatisfaction in teenage girls." This clarifies variables and the anticipated relationship, making it more testable and a stronger example of a research hypothesis.
Untestable Predictions
Another common error is proposing an untestable hypothesis. "People are happier when they have a purpose in life" is difficult to test because "happiness" and "purpose" are subjective. A more testable version would use measurable indicators: "Individuals reporting a strong sense of purpose also score higher on standardized well-being scales." This uses concrete, measurable variables.
Ignoring Existing Literature
Strong hypotheses are grounded in existing research. Failing to review relevant literature can lead to redundant research or easily disproven hypotheses. For example, proposing a hypothesis about the link between smoking and lung cancer without acknowledging established research would be a significant oversight. Thorough literature review enables researchers to build upon existing knowledge and develop novel, informed hypotheses.
Confusing Correlation and Causation
Correlation does not equal causation. Two variables being related doesn't mean one causes the other. Observing a correlation between ice cream sales and crime rates doesn't mean ice cream causes crime. Hypotheses should avoid implying causation unless the research design specifically tests causal relationships, ensuring accurate interpretation and preventing misleading conclusions.
Overly Complex Hypotheses
While complex hypotheses involving multiple variables can be valuable, avoid unnecessary complexity. Overly complex hypotheses can be difficult to test and interpret. Start with simpler hypotheses, increasing complexity as needed for a more manageable research process. Building a solid foundation with simpler hypotheses allows for exploring more complex relationships later, increasing the likelihood of generating valuable research.
Conclusion
This post has highlighted the importance of hypotheses in research, from foundational principles to diverse examples across different fields. We've examined various types of hypotheses, including simple, complex, directional, non-directional, and null/alternative, demonstrating how each serves a specific purpose in addressing different research questions. We also provided a practical guide for formulating strong, testable hypotheses, emphasizing clarity, specificity, and grounding predictions in existing research. This empowers researchers to approach investigations with a robust roadmap, ensuring studies are focused, rigorous, and contribute significantly to their fields.
Key Takeaways for Effective Hypothesis Formulation
- Clarity is Paramount: A well-defined hypothesis clearly articulates the relationship between variables, preventing ambiguity and focusing the research process.
- Testability is Essential: Hypotheses must be empirically testable, meaning the variables and predicted relationships are measurable and observable, enabling data collection that supports or refutes the hypothesis. Real-world examples demonstrate this is crucial for valid conclusions.
- Grounded in Existing Knowledge: Robust hypotheses build upon prior research and theories. Literature review helps avoid redundant research and ensures hypotheses are informed by current knowledge.
- Avoid Common Pitfalls: Awareness of common mistakes, like confusing correlation with causation and creating untestable predictions, prevents undermining investigations and helps develop sound and feasible hypotheses.
By following these principles and learning from real-world examples, researchers can develop powerful hypotheses that drive impactful investigations and deepen our understanding of the world. For streamlined writing and enhanced research organization, consider Shy Editor, an AI-powered writing tool designed to elevate your research process. Learn more about Shy Editor and how it can empower your research endeavors.