- Published on
Guide to Data Analysis Methods in Research
- Authors
- Name
- Tony
- @shyeditor
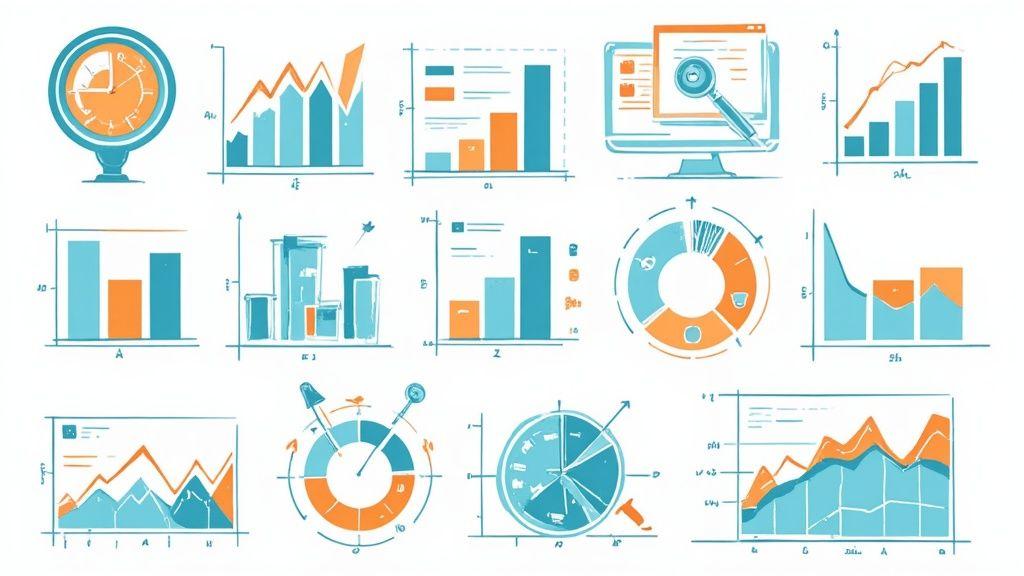
Breaking Down the Analysis Puzzle
Selecting the right data analysis methods is one of the most important decisions researchers face. This choice shapes how effectively you can answer your research questions and draw meaningful conclusions. Let's explore the key considerations that can help you confidently choose analysis methods for your next project.
Aligning Methods With Research Questions
Your research question should be the primary guide for selecting analysis methods. Think about what type of answer you're seeking. For instance, if you want to understand how advertising spending affects sales, you'll need quantitative methods like regression analysis to measure that relationship. But if you're interested in understanding why customers prefer certain products, qualitative methods like interviews would be more appropriate. Starting with a clear research question helps ensure your analysis approach fits your goals.
Quantitative vs. Qualitative: Choosing the Right Approach
The nature of your data and research goals determines whether you need quantitative analysis, qualitative analysis, or both. Quantitative methods work with numbers and statistics to find patterns - perfect for questions about "how many" or "how often," like analyzing customer purchase amounts or population demographics. Qualitative methods examine text, interviews and observations to uncover themes and meanings - ideal for understanding complex topics like employee satisfaction or product feedback. Each approach has distinct strengths for different types of research questions. Learn more in our article about Quantitative vs. Qualitative Research.
The Power of Combining Approaches
Many researchers find that using both quantitative and qualitative methods provides deeper insights than either approach alone. For example, a customer satisfaction study might use surveys to measure satisfaction scores across different segments, then follow up with interviews to understand the reasons behind those ratings. This combination of methods helps paint a complete picture by revealing both the "what" and the "why" of your research topic.
Overcoming Analysis Paralysis
With so many analysis options available, it's easy to get stuck trying to choose the perfect method. The key is to focus on your core research question and the type of data you have. Start by identifying methods that align with these fundamentals. Don't hesitate to consult with experienced researchers or statisticians for guidance. Remember that you don't need to use every possible technique - just the ones that will best answer your specific questions. Breaking the process into smaller steps and maintaining focus on your research goals will help you move forward confidently with your analysis.
Making Statistics Your Research Ally
Statistics play a key role in research by helping make sense of data and uncover meaningful insights. Understanding different statistical methods allows researchers to analyze their findings effectively and draw reliable conclusions. Let's explore how descriptive and inferential statistics work together to tell the complete story of research data.
Descriptive Statistics: Painting a Clear Picture
Think of descriptive statistics as the foundation for understanding your data. Just as an artist starts with basic shapes before adding detail, descriptive statistics give us the fundamental characteristics of our dataset. They show us things like typical values (mean, median, mode) and how spread out the numbers are (standard deviation, variance).
For example, when looking at customer satisfaction ratings, descriptive statistics tell an important story. A high average rating combined with minimal variation suggests consistent satisfaction across the board. But if there's high variation despite a good average, it could mean some customers are very happy while others are quite dissatisfied - a red flag worth investigating further. These basic measures help spot patterns that merit deeper analysis.
Inferential Statistics: Unveiling Hidden Relationships
While descriptive statistics summarize what we can observe directly, inferential statistics help us make educated guesses about larger populations based on our sample data. Through statistical tests, we can identify meaningful relationships between variables and determine if our findings are statistically significant rather than due to chance.
Regression analysis is a prime example. This technique examines how changes in one variable (like advertising budget) affect another (like sales revenue). Similarly, hypothesis testing lets researchers evaluate specific predictions about their data. For instance, a researcher might test whether a new teaching method actually improves test scores compared to traditional approaches.
Choosing the Right Statistical Test
Picking the appropriate statistical test depends on your research question, data type, and how that data is distributed. Here's a simple overview of common statistical tests and when to use them:
Statistical Test | Purpose | Data Type |
---|---|---|
T-test | Compare the means of two groups | Continuous |
ANOVA | Compare the means of three or more groups | Continuous |
Chi-square test | Analyze the relationship between categorical variables | Categorical |
Correlation | Measure the strength and direction of a linear relationship | Continuous |
While this list covers the basics, consulting with a statistician can help ensure you choose the most appropriate test for your specific needs. By understanding these core statistical tools, researchers can effectively analyze their data and present compelling findings that advance knowledge in their field.
Uncovering Hidden Patterns Through Exploration
After establishing a solid statistical foundation, researchers must take the next vital step: exploratory data analysis (EDA). This systematic approach helps turn raw numbers into meaningful discoveries by revealing patterns and relationships that might otherwise remain hidden. Like detectives piecing together clues at a crime scene, analysts carefully examine their data to uncover important connections and generate testable theories. Let's explore practical methods for diving deep into data analysis, going beyond basic charts to unlock the full power of visualization and pattern recognition.
Data Visualization
Good data analysis relies heavily on effective visualization techniques. Simple tools like scatter plots, histograms, and box plots can reveal relationships that remain invisible in raw data tables. For instance, a scatter plot might show a clear positive relationship between two variables that wasn't apparent before. But visualization isn't just about making attractive graphs - it requires choosing the right type of display for your specific data and research question. The key is using visual tools strategically to bring the story in your data to life and make patterns easy to spot.
Pattern Recognition
Beyond creating basic visualizations, EDA involves identifying complex patterns across multiple variables. This includes finding outliers that don't fit expected patterns, detecting natural groupings in the data, and spotting recurring sequences over time. For example, a marketing study might use cluster analysis to group customers based on their buying habits, leading to more targeted promotional strategies. Finding these patterns often combines rigorous statistical methods with the researcher's own subject matter expertise and analytical instincts.
From Exploration to Hypothesis: Generating Research Questions
One of the biggest benefits of EDA is how it sparks new research questions and hypotheses. By approaching the data with an open mind, researchers often notice surprising relationships they hadn't considered before. For example, a medical researcher studying a new medication's effects might discover an unexpected pattern suggesting a previously unknown side effect. This observation could lead to important new research questions about how and why this side effect occurs. This back-and-forth between exploration and hypothesis generation drives scientific progress forward.
Practical Applications of EDA
We can see EDA's impact across many different fields. Public health researchers use it to identify disease risk factors in large patient databases. Marketing teams apply it to better understand customer preferences and improve their products. Social scientists use EDA to analyze survey responses and social media data to understand human behavior patterns. This versatility in extracting insights from complex datasets makes EDA essential for researchers in virtually any field. By combining careful observation with systematic analysis, EDA helps unlock new discoveries that advance human knowledge.
Mastering Advanced Analysis Techniques
Once you've mastered basic exploratory analysis, it's time to dive into more sophisticated methods that can reveal deeper insights from complex datasets. These advanced techniques allow researchers to uncover subtle relationships and patterns that may not be immediately obvious. Let's explore some key advanced analysis approaches and see how they work in practice.
Regression Analysis
At its core, regression analysis helps us understand how different variables influence each other. For instance, a business analyst might use linear regression to figure out exactly how advertising budgets affect sales numbers. This not only shows the connection between spending and revenue but can predict future sales based on planned ad costs. While basic linear regression works for simple relationships, other types like logistic regression handle yes/no outcomes, and polynomial regression can map curved relationships between variables.
Cluster Analysis
Think of cluster analysis as a way to sort data points into natural groupings based on their shared traits. A retail company might use this to segment their customer base by analyzing purchase history, demographics and preferences. This could reveal distinct groups like bargain hunters, luxury shoppers or tech enthusiasts. The business can then craft targeted marketing campaigns and product offerings for each segment. For example, an online retailer might adjust product recommendations based on which customer cluster someone belongs to.
Pattern Recognition
Pattern recognition helps spot meaningful trends that basic statistics might miss. Financial companies use these techniques to catch fraud by identifying unusual transaction patterns that differ from a customer's normal behavior. In healthcare, pattern recognition algorithms scan medical images to spot early signs of disease based on subtle visual indicators. The real power comes from finding these hidden patterns automatically, without relying on manual review.
Choosing the Right Advanced Method
Picking the best analysis method depends on three key factors: your research question, your data type, and what you want to learn. If you need to predict outcomes, regression analysis is your best bet. For grouping similar items together, cluster analysis works well. Complex pattern detection calls for specialized recognition algorithms. Often, combining multiple methods gives the most complete picture. Before diving in, take time to understand each technique's strengths and limitations. For complex projects, consulting with statistics experts can help ensure you're using the right tools for your specific needs.
Building Credible Research Through Validation
While data analysis methods offer powerful ways to uncover insights, the real strength of research comes from validating those findings properly. Just as a solid foundation supports an entire building, thorough validation ensures research conclusions can withstand scrutiny. Let's explore the key techniques researchers use to make their findings reliable and reproducible.
Testing Assumptions and Handling Outliers in Data Analysis Methods
Most research methods rely on specific assumptions about the data being analyzed. For instance, when using linear regression, researchers must verify there's a linear relationship between variables and that residuals follow a normal distribution. Testing these assumptions is essential - if they don't hold true, the results may be misleading or incorrect. Consider how non-normally distributed residuals could signal outliers or non-linear relationships that require different analytical approaches. Similarly, researchers need strategies for handling outlier data points that deviate significantly from typical values. Common approaches include data transformation, careful trimming, or winsorizing techniques, chosen based on the specific data and analysis needs. This systematic approach helps ensure the analysis captures genuine patterns rather than being skewed by extreme values.
Ensuring Reproducibility: A Cornerstone of Credible Research
For research to be truly credible, other researchers should be able to replicate the study and reach similar conclusions using the same methods and data. This means providing detailed documentation of every step - from specific analysis techniques to software versions and data preprocessing procedures. When other researchers can follow your exact process and verify the results independently, it builds confidence in the findings. Making data and code publicly available (where appropriate) further supports transparency. This open approach allows the broader research community to verify and build upon your work, strengthening the overall credibility of the findings.
Maintaining Data Integrity
Keeping data accurate and reliable throughout a research project requires careful attention at every stage. This starts with using proven data collection tools and implementing thorough quality checks during data entry. Regular verification helps catch inconsistencies early, while secure storage and backup procedures protect against data loss. By focusing on data quality from collection through analysis, researchers ensure their conclusions rest on solid evidence. When combined with proper validation techniques, this careful approach to data handling creates research that others can trust and use confidently to advance knowledge in the field.
Communicating Your Findings for Maximum Impact
The success of data analysis depends heavily on how well you communicate the results. After investing significant time and effort in collecting and analyzing data, you need to present your findings in a way that resonates with your audience - whether they're academics, professionals, or the general public. This requires choosing effective visualizations, building a clear narrative around your statistical results, and addressing potential questions head-on.
Visualizing Your Data: Choosing the Right Chart for the Right Story
Our brains process visual information much faster than text. Selecting appropriate visual formats helps convey complex data quickly and memorably. For time-based trends, line graphs work well. When comparing data distributions across groups, box plots or histograms are often better choices. Pie charts effectively show parts of a whole, while scatter plots reveal relationships between variables. Research by the Nielsen Norman Group shows that people remember information 80% more accurately when it's presented visually versus text alone. This highlights why choosing the right visualization format is so important for communicating your analysis.
Crafting Your Narrative: Turning Numbers Into Insights
Numbers and charts alone aren't enough - they need context through storytelling. Begin by clearly stating your research question and what you expected to find. Guide your audience step-by-step through what the data shows and what it means for your research goals. Use clear language that your audience will understand. For example, instead of statistical jargon like "p < 0.05," explain that "these results are unlikely to have occurred by chance." Make sure to connect your findings to real-world implications. What practical impact could your research have? A strong narrative transforms raw data into meaningful insights.
Addressing Potential Criticisms
Directly addressing limitations and alternative interpretations makes your research more credible. For instance, if you worked with a small sample size, explain how you accounted for this or why your findings are still valid. If there are other possible ways to interpret the data, discuss them and provide evidence for why your interpretation makes the most sense. Taking this proactive approach builds trust in your analysis methods and helps your findings gain acceptance.
Presentation Best Practices: From Slide Decks to Publications
Different formats require different presentation approaches. For talks, use clear slides with impactful visuals. For papers, follow standard formatting and provide detailed methods. For blog posts, focus on accessibility and key takeaways. Whatever the format, tailor your message to fit both the medium and your specific audience to achieve maximum impact.
Ready to improve your research writing? Shy Editor provides an AI-powered writing environment to help communicate your research clearly and effectively. From checking grammar to organizing content, Shy Editor helps streamline your workflow so you can focus on building compelling narratives around your findings. Start writing smarter today with Shy Editor.